Introducing BharatBench: Making AI Understand India
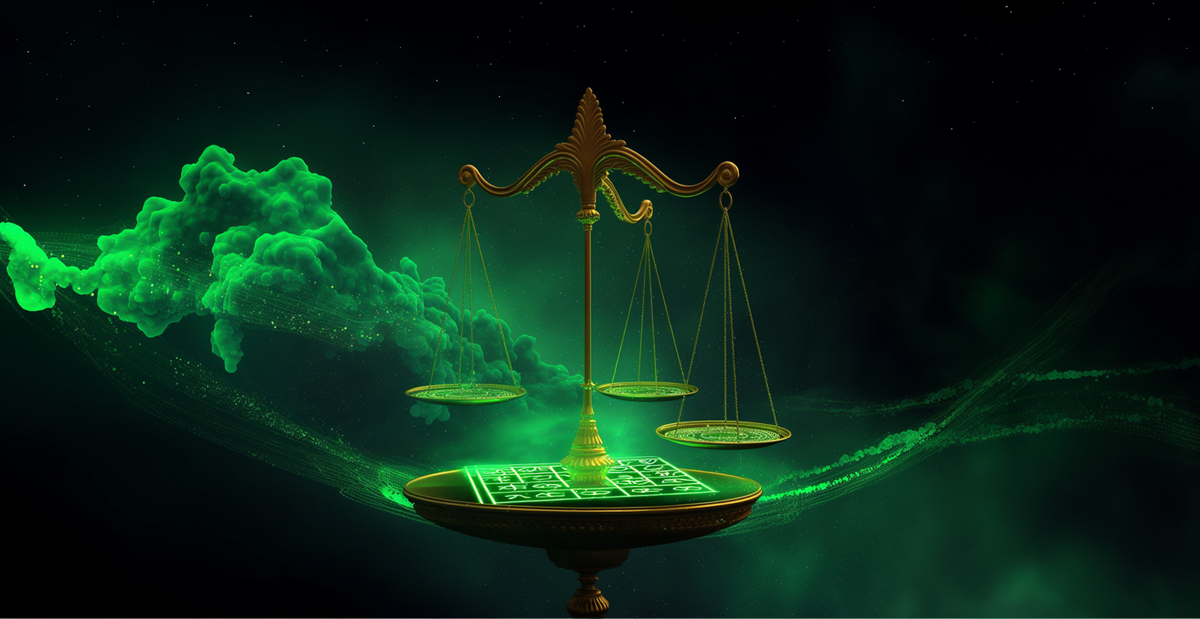
Have you ever wondered if the AI you use every day truly understands India and its diverse culture? Most AI models are trained primarily on English, Chinese, or European languages, which means they might miss the nuances of Indian languages and cultural contexts. That's where BharatBench comes in.
What is BharatBench?
BharatBench is a Comprehensive, Multimodal, Multilingual, Multi-task Indic benchmark. It is a special evaluation tool designed to test how well AI models understand and perform in Indian languages and cultural contexts. Think of it as a report card for AI, but specifically for its ability to work with the diverse needs of India. It helps us see which AI models are truly ready for the Indian market and which ones need improvement.
Why is BharatBench Important?
• Addresses the gap: Many current AI models don’t work well with Indian languages. They often struggle with understanding the different ways people speak and the cultural context. BharatBench helps highlight these issues.• Lack of a Comprehensive, Multimodal, Multilingual, Multi-task Indic benchmark: Existing benchmarks in English and Chinese, such as MMLU, ARC, TruthfulQA and others, do not adequately capture the unique linguistic and cultural nuances of India. There is a specific need for a benchmark that addresses the diversity of India.
• Ensures inclusivity: With over a billion people speaking various Indian languages, it's important that AI is inclusive. BharatBench ensures that AI models are evaluated on their performance with these diverse languages and cultures.
• Improves AI quality: By using BharatBench, researchers and developers can create better AI models that are specifically optimized for the Indian market. This means more accurate and relevant AI for everyone in India.
• Promotes fair evaluation: BharatBench was created because current AI evaluation methods don't always work well for Indian languages. It's important to have specific tools that can test AI fairly in these contexts.
What Does BharatBench Evaluate?
BharatBench tests AI models across several key areas:
• Language Understanding: It checks how well AI models can understand and generate text in Indian languages. It uses various tasks including things like comprehension, translation and grammar correction. This includes seeing if models understand Indian cultural contexts, such as the importance of festivals and traditions.
• Distributional Representations: Assesses embedding models for sentence retrieval, evaluating their ability to handle Indian languages, contexts, and large documents
• Visual Understanding: It assesses how well AI understands images related to India. This includes things like recognizing Indian festivals, art, historical sites, and more. It can also read text in images, like old books and handwritten notes.
• Speech Understanding: BharatBench evaluates how well AI can understand spoken Indian languages. This includes transcribing speech to text and translating it accurately.
How Does BharatBench Work?
BharatBench uses a collection of real-world examples and datasets specifically designed to represent the linguistic and cultural diversity of India. This ensures that AI models are evaluated on their performance with real-world use cases. The models are tested across multiple Indic languages, including Hindi, Marathi, Tamil, Telugu, Kannada, Gujarati, Bengali, and Malayalam and others. The framework also includes several evaluation techniques, including looking at how well AI models can perform in specific language tasks like:
• Indian Cultural Context (ICC): This assesses AI’s understanding of Indian customs, traditions, and social practices.
• Multi-turn comprehension: This evaluates the model’s ability to understand and interpret passages of text from varied domains.
• Multi-turn Translation: This evaluates the model’s ability to translate accurately between languages, including the handling of idioms and colloquial phrases.
• Text classification: This tests how well AI can classify text into different categories, like sentiment, topic, intent, and language.
• Grammar Correction: It checks the AI's ability to identify and correct grammatical errors in Indic languages.
Key Findings
• BharatBench uses real-world scenarios and data to assess the AI landscape in India.
• AI models that are trained specifically for Indian languages tend to perform much better than generic models.
• Some AI models are better at certain tasks than others. For example, some may be better at grammar correction while others might excel at translation.
• Krutrim LLM series, models trained specifically for Indic languages, have shown very promising results across various tasks
• Vyakyarth, a new embedding model, has shown high performance in understanding the nuances of multiple Indic languages
• For visual understanding, Chitrarth outperforms other models in understanding images, showing it is better at understanding Indian cultural contexts
• Shabdarth, a custom OCR model, has shown good performance in reading Indic content, including old books with complex scripts.
• Custom speech models perform better than generic models, indicating a need for models specifically designed for Indic languages
• Need for Better Evaluation: Existing evaluation methods are often biased towards high-resource languages. There is a need for custom multilingual evaluators which BharatBench addresses.
Results
Language | MuRIL | IndicBERT | Jina-Embeddings-V3 | Vyakyarth |
---|---|---|---|---|
Bengali | 77.0 | 91.0 | 97.4 | 98.7 |
Gujarati | 67.0 | 92.4 | 97.3 | 98.7 |
Hindi | 84.2 | 90.5 | 98.8 | 99.9 |
Kannada | 88.4 | 89.1 | 96.8 | 99.2 |
Malayalam | 82.2 | 89.2 | 96.3 | 98.7 |
Marathi | 83.9 | 92.5 | 97.1 | 98.8 |
Sanskrit | 36.4 | 30.4 | 84.1 | 90.1 |
Tamil | 79.4 | 90.0 | 95.8 | 97.9 |
Telugu | 43.5 | 88.6 | 97.3 | 97.5 |
Table: Performance of Indic Embedding models across different languages
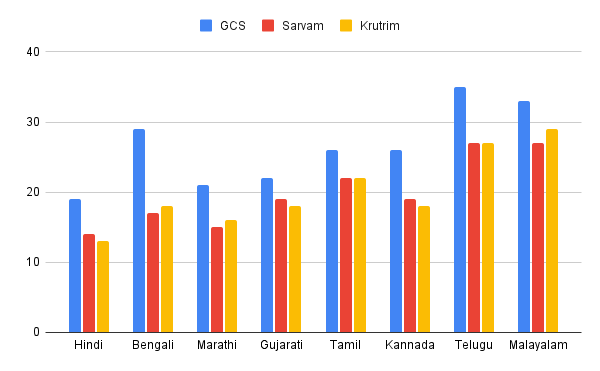
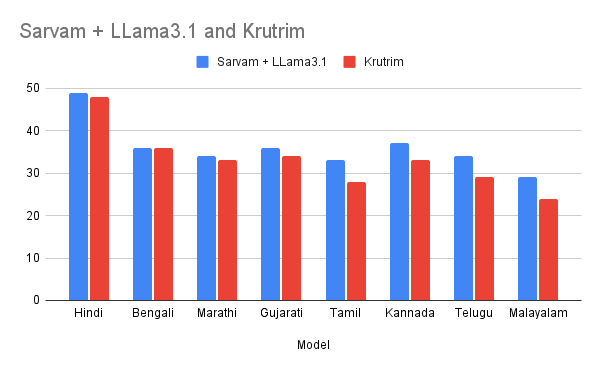
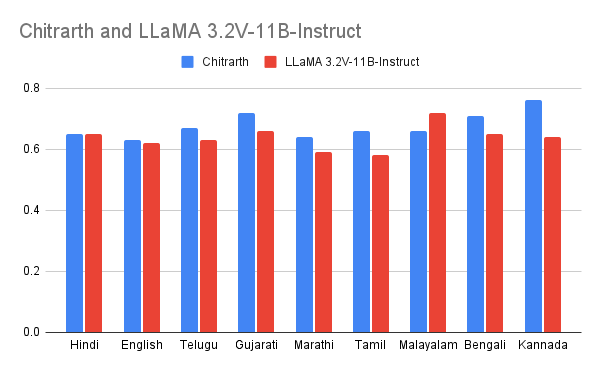
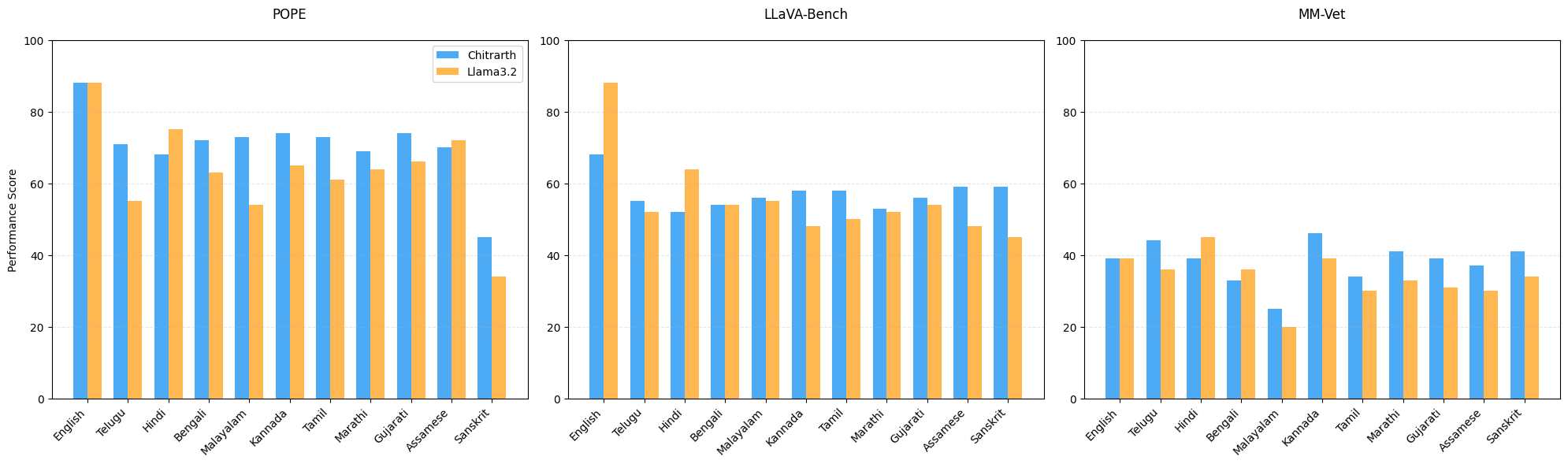
Figure: Character Error Rate (CER) at the top and Word Error Rate (WER) below (lower is better). Shabdarth model performs better against GPT-4o and GCP for the BharatBench OCR dataset
Table: Performance of different models on BharatBench NER tasks. We report 5-shot accuracy for the NER task.
Table: Performance of different models on BharatBench text classification task. We report 0-shot accuracy for the Text classification task.
Table: Performance of different models on BharatBench ICC task. We report 0-shot BERT Score.
Why This Matters:
◦ Better AI for India: BharatBench is a step towards creating AI that better understands and serves the diverse population of India.
◦ More Inclusive AI: The framework promotes inclusivity in the AI ecosystem by focusing on underrepresented languages and cultures.
◦ Informed Decisions: It helps businesses and researchers choose the best AI models for Indian use cases.
The Future of BharatBench
BharatBench is in its nascent stage and will continue to expand its evaluation capabilities, including adding more languages, prompts, and tasks. It is an important step toward building AI that is inclusive and relevant to India. By continuing to refine and use BharatBench, we can ensure that AI works for everyone.
Conclusion
BharatBench is a critical tool for evaluating and improving AI models for Indian languages and culture. It’s a step toward building more inclusive and effective AI that can serve the diverse needs of India.
Please check out our technical report for a more detailed discussion.
Authors: Shubham Agarwal, Abhinav Ravi, Rajkiran Panuganti, Sharath Adavanne, Ashish Kulkarni, Hareesh Kumar, Chandra Khatri